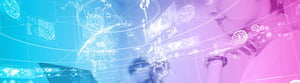
From Digital Twin Vision To Reality: How Companies Can Gain Business Intelligence from Digital Twins
Digital transformation has never moved so fast. The disruption caused by the pandemic has forced companies to roll two years’ worth of digital transformation into a handful of months. The vast majority of companies plan to grow investments in digital initiatives and pilot more Industry 4.0 technologies. With the rapid pace of digital transformation, it’s no surprise that it comes at a steep cost: to the tune of $6.8 trillion by 2023 as companies build on their existing strategies.
But as the frantic pace of digital transformation continues to accelerate, manufacturers face mounting pressure and uncertainty. The average digital maturity is at a mere 39 percent, and 83 percent of companies have low maturity in digital capabilities that drive significant value. The rapid change and complex tools available today make digital transformation one of the most promising and most frustrating initiatives in today’s manufacturing landscape.
The Value Of A Digital Twin
Perhaps the most well-known digital transformation archetype lies with the digital twin. Accenture calls digital twin technology a top trend for 2021, with 42 percent of companies either scaling or experimenting with it. Digital twins are proving to bring significant value:
- 87% of executives agree digital twins are becoming essential to their ability to collaborate in strategic ecosystem partnerships.
- 31 percent of companies use digital twins to improve safety, such as with remote asset monitoring
- Aerospace companies see up to a 75% reduction in design cycle time, up to 8x reduction in testing, and 40% reduction in maintenance costs
The average digital maturity is at a mere 39 percent, and 83 percent of companies have low maturity in digital capabilities that drive significant value.
Despite the progress so far, implementing the technology to enable a digital twin is no small feat. It’s difficult to even nail down a common understanding. Consider the wide spectrum of how a digital twin is described by the industry’s leading experts:
- A near-real-time digital image of a physical object or process that helps optimize business performance.
- A living model in 3D
- A management model to improve enterprise decisions about something specific
- A software design pattern that represents a physical object to understand the asset’s state, respond to changes, improve business operations, and add value
- An intelligent, collaborative, interactive, immersive, and fully contextual representation within the enterprise
If all these definitions feel overwhelming, there are a few underlying themes to consider. First, what the digital twin is: a 3D, digital representation that continuously processes enormous amounts of data from real-world products. A digital twin is an amalgamation of as-designed, as-built, and as-maintained information. But the true value of a digital twin is its ability to give companies business intelligence to make better decisions about their products:
1) Link the physical and digital worlds in real-time. Today, there are discrepancies between as-designed and as-built documentation. A digital twin stores aggregate information throughout the lifecycle, allowing companies to access all requirements, concepts, validations, estimated costs, suppliers, service lifecycles, and field data.
2) Drive product quality and innovation. All the information gathered from connected sensors and endpoints streams together to give insight on a product’s performance. This in turn allows manufacturers to identify areas for improvement. For example, a company can use a digital twin’s field data to understand how environmental elements (such as climate or terrain) impact a part’s expected performance. 63 percent of enterprises expect to achieve financial payback in 3 years for IoT projects.
3) Gain visibility and analytics for continuous improvement. Sensor data also gives manufacturers the ability to predict and plan for maintenance needs. A company can discover when a component starts to lose performance and perform preventative maintenance before the part physically fails. In fact, Gartner predicts that predictive asset management can lead up to 40 percent cost savings by 2022.
4) Connect different teams for real-time decision-making. 92 percent of organizations can trace miscommunication as the root cause of product issues. Of those organizations, 30 percent experience more than 10 issues per month due to miscommunication. Digital twins give engineering, manufacturers, sales, service, marketing, and other disparate teams a system of engagement to understand aggregate real-time data from multiple systems. This helps teams get the right view of their data and avoid miscommunication pitfalls.
The true value of a digital twin is its ability to give companies business intelligence to make better decisions about their products.
5) Lower costs. Consider a culmination of consistent documentation, increased quality, data analytics, and better communications. Manufacturers stand to save millions—even billions—of dollars and countless hours of time thanks to greater product understanding.
A Digital Twin’s Journey
Digital twins give context and insight into each stage of the product lifecycle, including design, build, and in-the-field usage.
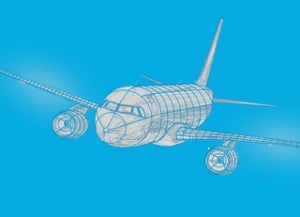
Engineering and design teams create a product’s design, including requirements and simulation results to understand how a product will perform. This “as-designed” information kicks off the digital twin’s journey.
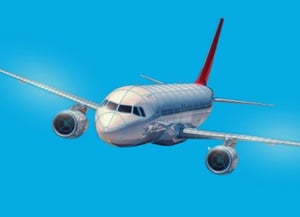
As manufacturing teams turn the design into reality, they quickly add any replacements or changes to the digital twin to record “as-built” information.
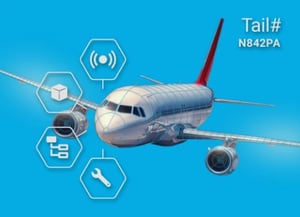
Once a product is in the field, a multitude of sensors gather information about the product’s performance. This information streams back into the digital twin to record “as-manufactured” data.
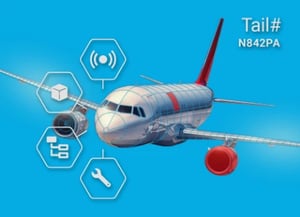
Companies take design, build, and performance data to understand what is needed for “as-serviced” information and provide learning and predictive analytics. With the right technology in place, a digital twin can understand information from sensors and relate it to the as-designed and as-built information to gain insight on improving future iterations.
3 Challenges With A Digital Twin
So if digital transformation and harnessing the power of digital twins are proven to save companies millions of dollars and man-hours, skyrocket product quality, and escalate cross-enterprise collaboration - why are companies still struggling to implement?
1) An Overwhelming Amount of Data
When moving towards a digital transformation strategy, many companies don’t fully prepare for the sheer volume of data that will come in. All the combined information from product designs, sensor readings, service reports, and other critical documentation is staggering. Real-time data alone represents 15 percent of all digital content globally, and it’s expected to hit 30 percent by 2025.
In fact, IoT sensors and devices are expected to create over 90 zettabytes of worldwide data by 2025 (one zettabyte is a trillion gigabytes). If that feels like a nebulous number, consider calculations made by senior vice president of IDC: storing 90 ZB of data onto BluRay discs would create a stack that would get you to the moon nearly 12 times.
Tip for success: Prepare and plan for the amount of data your company will receive. Investigate AI, edge technologies, machine learning solutions to help manage the influx of information. With the right solutions in place, companies can get clear indicators of actionable insights.
2) Silos Are (Still) Hard To Break Down
Despite the digital technology and tools at our fingertips, engineering teams are still relying on outdated methods to gather information. One report showed that the most common way of finding and accessing engineering information was to physically collect information from person to person. And over half of engineers manage information spread across multiple systems. Only half of companies that have digital twins use their twin to serve more than one team. And, organizations create multiple digital twins of different components that make up a single product. Only a quarter of companies actually integrate multiple digital twins for a holistic product into one single source.
We’ve already determined that the true purpose of a digital twin is to amalgamate documentation and information from all teams and steps throughout a product's lifecycle from cradle to grave. If that’s true, why do most companies create multiple digital twins at a component level or rely on directly talking with engineers to gather information? Although the promise of digital transformation brings teams together, the idiom “old habits die hard” has never felt more real as companies contend with decades-old processes that separate functional departments.
Tip for success: Much of cross-functional barriers are rooted in two areas: cultural change and access to the correct technology. Rather than trying to grant access to different systems of record, integrating a system of engagement gives teams the ability to access the information they need without sifting through different record systems. This helps to bridge cultural changes without struggling with different technology systems.
3) Expected Automation Vs. Human Requirements
Many companies and teams relate digital transformation to automation. Although the two frequently go hand-in-hand, industry expectations have perceived machine learning, predictive analytics, and automation as meaning the same thing as digital transformation. As such, companies don’t always account for how much is still needed on the human application side.
Stakeholder interactions, applying expertise, problem-solving, and other collaboration activities account for nearly half of all the work done in manufacturing companies. Very little of this work can be automated. Without fully understanding and planning for this, companies (and engineers in particular) may be quick to dismiss digital workflows as a failure because it didn’t automate certain pieces of the workflow.
Tip for success: Clearly differentiate automation processes from an overall digital transformation strategy. Remember that the goal of digital transformation and digital twins is not to automate or remove workflows, but instead to provide the necessary business intelligence to focus work on more strategic and valuable outputs. This breaks down silos and helps boost collaboration across different teams.
Phasing In Digital Transformation Changes
Regardless of whether your company is undergoing a formal digital transformation, every company is experiencing digital change of some kind. To discern a successful from mediocre implementation, companies have to be diligent and thoughtful in their transformation. Every company’s approach will look slightly different, but there are four general phases a manufacturer should undergo.
1) Establish Your Strategy
Begin by establishing your strategy both in terms of the workflows and processes needed. Once your company has a good foundation for a process strategy, then consider a technology plan. Make sure your plan includes a platform for dashboards and views to understand your progress. Factor in configuration and change management as well to determine if your strategy can scale up. Establish how you will measure KPI’s and what metrics are most important to you so you can clearly identify the success or failure of the strategy.
Here are a few guiding questions to get you started:
- What is your specific goal?
- What outcomes will tell you that you’ve achieved that goal?
- Which project or process will you use to test that goal?
- How will this impact greater company initiatives?
The true purpose of a digital twin is to amalgamate documentation and information from all teams and steps throughout a product's lifecycle from cradle to grave.
2) Plan A Pilot Test
Although a pilot test itself is almost always implemented, many companies overlook or downplay the importance of the upfront preparation needed. The nature of the manufacturing industry is to continuously release “cool” new tools that promise to provide value. New tools can require long and expensive process overhauls, and some are agile and cost-effective. Companies should first determine which type of tool is best for them based on the promised return, start-up time, and cost. Combine this mindset with ensuring your tools truly address a pain or need in your company. The more specific you can be in identifying a particular use case or problem you want to solve, the more successful the technology implementation will go.
Here are a few guiding questions to get you started:
- What workflows are currently in place that may be disrupted?
- Who will be directly impacted? Who will be indirectly impacted?
- Do we have the capability to integrate AI or machine learning to help manage the influx of information? How do we implement them?
- What KPI’s are most important to us? How will we measure the success of the pilot?
3) Measure & Monitor
Once the pilot test is implemented, closely monitor the progress and results. Analyze how well your results match to your predetermined KPI’s. It’s just as important to also check in with the affected teams to understand how the pilot test is impacting their workflows and perceived quality of work. Throughout the pilot test, maintain agility and flexibility to quickly make incremental changes.
Here are a few guiding questions to consider:
- Are the KPI’s what you expected?
- How is the team doing at adapting workflows?
- What results are unexpected, both in a negative and positive way?
- Where are the areas for improvement?
4) Scale When You’re Ready
If you’ve done a thorough job of identifying and measuring metrics and KPI’s, you will know if the pilot was successful enough to proliferate throughout your organization. Before releasing the new technology and workflow processes to the wider team, it is imperative to begin this process over again.
Clearly identify the greater company outcomes, strategy, process, and purpose for scaling. Communicate the value of the change to all affected employees. Process change is always a challenge, and implementing the huge change inherent in digital transformation can create friction. Clear communication helps to manage cultural change and helps your team rally behind your cause.
How to Use a Digital Twin for Business Intelligence
Let’s put this phased approach into action. For the purposes of this example, let’s consider an auto manufacturer with a global presence who does not yet have a formal digital transformation process and is in early stages of adoption.
1) Establish Your Strategy
A common problem that many large-scale manufacturers face today is getting 3D models and product data to anyone who needs it. Typically, companies need to translate files into multiple different types (such as native formats, common formats, stripping to PDF, or screenshotting) so that downstream teams can access the information they need. This puts a heavy burden on the engineering department, and opens the door for miscommunication, loss of feedback, orphaned data, and possible IP risk.
In our example, let’s say the automotive manufacturer wants to tackle this challenge head-on. Their current process requires a dedicated team to transform data into usable formats for suppliers, procurement, manufacturing, service teams, and customers. This process can take weeks or months of valuable development time, and there are minimal feedback loops to update documentation during as-built and as-serviced.
To begin establishing their strategy, the auto company may use these guiding questions:
- What is your specific goal? Reduce two weeks in the engineering and product development lifecycle. We believe a targeted use case to reduce overall timeline is to minimize or eliminate time spent on data transformation and translations.
- What outcomes will tell you that you’ve achieved that goal? Shorten our development cycle by a minimum of two weeks and cut data transformation steps in half.
- What project or process will you use to test that goal? We will test this process on one of the necessary changes for our 2021 model of our standard 4-door sedan: adjusting a transmission to improve fuel economy and prolong the time between failures. We have selected this because the required change should be fairly simple and we have the agility to pivot back to old processes quickly if we need.
- How will this impact greater company initiatives? By creating a single source of truth through a digital twin, we can gain business intelligence into our products for future iterations and improvements. This will help boost collaboration and adopt a system of engagement instead of our current monolithic data architectures.
The goal of digital transformation and digital twins is not to automate or remove workflows, but instead to provide the necessary business intelligence to focus work on more strategic and valuable outputs.
2) Plan A Pilot Test
Now that the automotive manufacturer fully understands the problem and specified goals, they begin researching possible technology solutions and how they will change processes. After a stringent research and vetting process, they decide to pilot the Vertex platform due to its “system of engagement” approach to vastly improve the 3D user experience, lower cost, eliminate hardware requirements, simplify data sharing without the need for translations, and easily and quickly scale the pilot if it is a success. Leadership teams work closely with Vertex to identify a clear implementation and support process throughout a phased proof of concept.
The manufacturer decides to implement a pilot test to harness the power of a digital twin to collect and share product information in a single source of truth. This might be what their response to the guiding questions could look like:
- What workflows are currently in place that may be disrupted? The data transformation process will either be eliminated or drastically reduced. There will be a new process from engineering to share information with the necessary parties.
- Who will be directly impacted? Who will be indirectly impacted? Engineering and the data transformation team will be highly impacted. We anticipate additional impacts for procurement, manufacturing, and service teams.
- Do we have the capability to integrate AI or machine learning to help manage the influx of information? How do we implement them? By implementing different sensors in our products on the factory floor and out in the field, we can understand heat outputs and gear speeds that indicate fuel economy impacts and failure resistance. Vertex’s system of engagement will provide an overview of the necessary data we collect from our systems.
- What KPI’s are most important to us? How will we measure the success of the pilot? The most critical KPI’s to us include shortening the engineering and product development lifecycle in addition to improving the transmission rate’s heat outputs and failure rates. We will compare current data with the data we collect in the pilot to clearly understand the effect Vertex has on our workflows and product outputs.
3) Measure & Monitor
Prior to the implementation, the auto manufacturer takes a few weeks to fully determine current time spent and mapping out a typical process for data translation. This necessary step provides a baseline for understanding how Vertex impacts their workflows.
Over the course of the next few days, the engineering and data translation personnel are quickly onboarded and begin using Vertex to share product design changes on the transmission. Operations teams develop easily access the Vertex system of engagement to track time spent on design work. Field tests measure the impact of the transmission changes. The manufacturer remains agile and flexible and makes small adjustments over the course of the proof of concept to adapt what they initially planned for against the actual execution.
At the conclusion of the proof of concept, the automotive leadership team reviews the KPI metrics established at the start of the proof of concept. Using the guiding questions above, they determine:
- Are the KPI’s what you expected? We have seen significant success in shortening the time spent between design iterations based on real-time feedback from the field. We have also eliminated most costs related to design re-work and have shortened upfront design time by several weeks.
- How is the team doing at adapting workflows? The engineering team believes this to be a vast improvement from previous workflows. The data translation team has been transitioned to other critical activities due to the success of the platform.
- What results are unexpected, both in a negative and positive way? We have been able to utilize the platform with procurement, suppliers, service, and manufacturing departments. We have improved maintenance involvement, shop floor instructions, and vendor relations. With additional work, we could use this as our digital twin implementation.
- Where are the areas for improvement? Overall, we were very pleased with the capabilities of Vertex’s system of engagement and believe we could improve other steps in our design lifecycle.
Process change is always a challenge, and implementing the huge change inherent in digital transformation can create friction. Clear communication helps to manage cultural change and helps your team rally behind your cause.
4) Scale When You’re Ready
Before scaling to the broader organization, the automotive manufacturer pulls the results of the pilot test into an easily digestible report to provide to management across the enterprise. Teams host internal meetings to clearly communicate new processes and benefits to the current workflow. Due to the transparency of the leadership team and value in the pilot results, the team rallies behind the change and begin to implement Vertex throughout their organization.
Managing Digital Challenges With 3D Visualization
Recall the three digital challenges discussed earlier in this white paper. Clearly defining a plan, strategy, and KPI’s enable teams to manage many of the challenges in digital transformation and ultimately provide greater business intelligence. Vertex was designed to minimize the pains in digital transformation:
- An Overwhelming Amount of Data. With our 3D visualization platform, companies can access the right data when needed and align it to the 3D visuals available, gaining the full value of their current systems.
- Silos Are (Still) Hard To Break Down. Vertex brings fully interactive 3D experiences into purpose-built applications for any team, workflow, or purpose.We enable manufacturers to take full advantage of their current technology investments by unlocking 3D models and product data for downstream teams.
- Expected Automation Vs. Human Requirements. With the Vertex platform, teams connect to product data for applications in design, supply chain, manufacturing, sales, and service to easily and intuitively make decisions and consume mission-critical data.
If you’re interested in learning more about how Vertex enables 3D digital twins quickly and easily anywhere in your organization, get in touch with us at info@vertex3d.com.